Stephen Wolfram and Taleb on AI and decision making processes
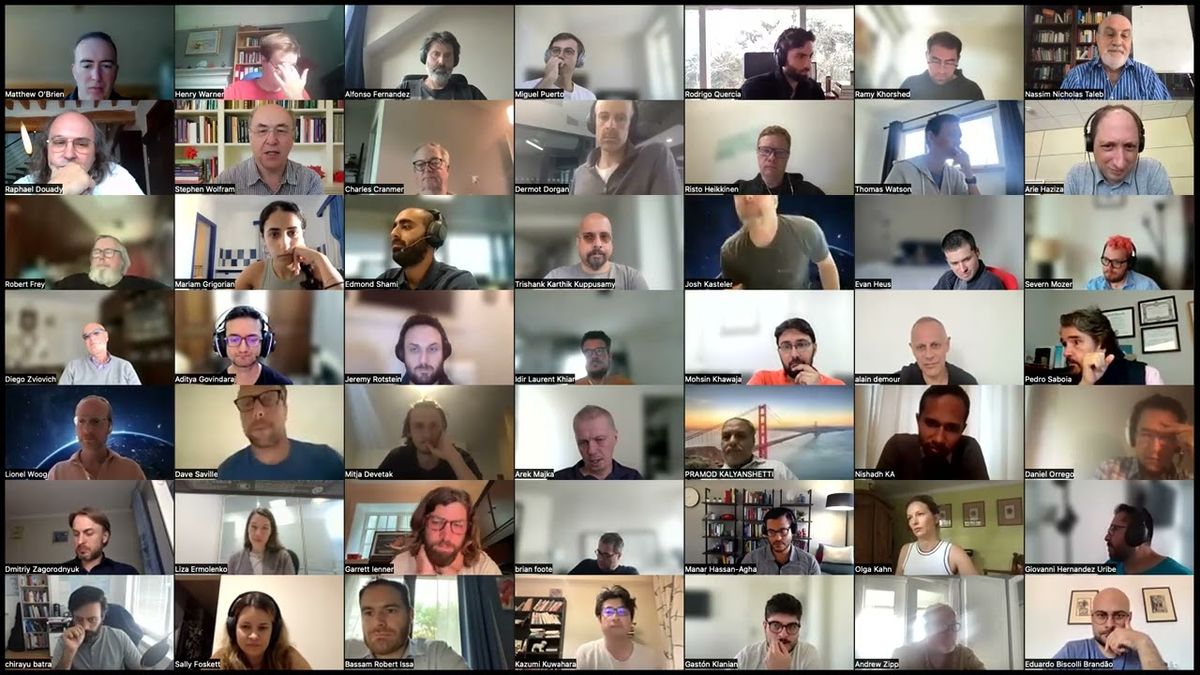
This video captures an intriguing discussion featuring Stephen Wolfram at the RWRI 18 (Summer Workshop).
The discourse delves into the complexities of artificial intelligence (AI), specifically focusing on language models like Chat GPT, and the challenges of integrating AI into decision-making processes.
It also explores the responsibility and ownership of AI systems and the potential implications on society.
Complexity of Defining AI Behavior
Determining principles for AI behavior proves challenging due to the lack of consensus and human inconsistency.
However, establishing these principles is crucial for responsible AI use, necessitating ongoing discussions and debates.
Challenges with Statistical Models
Statistical methods such as Chat GPT may struggle with outliers and inconsistencies.
This could potentially lead to rigid designations and inconsistent responses, emphasizing the need for more robust and flexible models.
Ownership and Responsibility of AI Systems
The ownership and responsibility for AI systems is currently unclear.
As AI systems are owned and developed by companies, it distinguishes their ownership structure from individuals or other entities.
In the future, AI systems may mimic the legal and ethical frameworks of corporations, suggesting a shift in how we perceive and manage AI systems.
Balancing AI Freedom and Constraints
Striking a balance between the freedom of AI to compute and discover, and the need for constraints and predictability, is a challenge.
This is especially true when dealing with computational irreducibility, signifying a complex tug-of-war between control and exploration.
AI in Decision-Making Processes
AI is increasingly woven into decision-making processes.
It’s imperative to consider how these decisions are made and their implications, underlining the need for thoughtful integration of AI in decision-making systems.
Unearthing Semantic Grammar
Language models like Chat GPT can reveal a higher level semantic grammar, which allows for more meaningful sentence construction beyond mere noun-verb relationships.
This discovery points to a new layer of structure within language.
Human Advantage in Error Estimation
Humans inherently understand error rates and can make estimates with asymmetric errors.
This ability to adjust error rates in different directions is currently lacking in AI systems like Chat GPT, underscoring a clear distinction between human and AI calculations.
Insofar as AIS can be owned, can be made by companies… the sort of structure of who’s really responsible looks a bit different. – Stephen Wolfram
Trust and Skepticism in AI
Trusting AI warrants caution and skepticism.
Both AI systems and humans can make unpredictable decisions due to computational irreducibility, necessitating a careful approach towards trusting AI.
Risk Mitigation in AI
Risk in AI can be mitigated by incorporating multiple systems, much like having multiple judges in decision-making.
This instills confidence in the overall outcome and reduces dependence on a single system.
Language has kind of a higher level semantic grammar that allows one to put sentences together in a meaningful way. – Stephen Wolfram
The Need for Redundancy
Incorporating layers and redundancy in decision-making processes can significantly reduce risks, minimize the impact of errors or biases, and increase the likelihood of a favorable outcome, validating the need for robust decision-making structures.
Balancing Power and Diversity in AI
Harmonizing the power of AI with diversity and creativity in decision-making is vital to prevent stifling innovation and limiting the emergence of new ideas, emphasizing the need for a broad and inclusive approach.
The Art of Writing Prompts for AI
Writing effective prompts for AI systems requires expository writing skills.
The formatting choices and instructions can influence the AI’s response, but it may not always yield the desired result, highlighting a gap between human intentions and AI interpretation.
Comments ()