The future of robots and perception systems with synthetic data
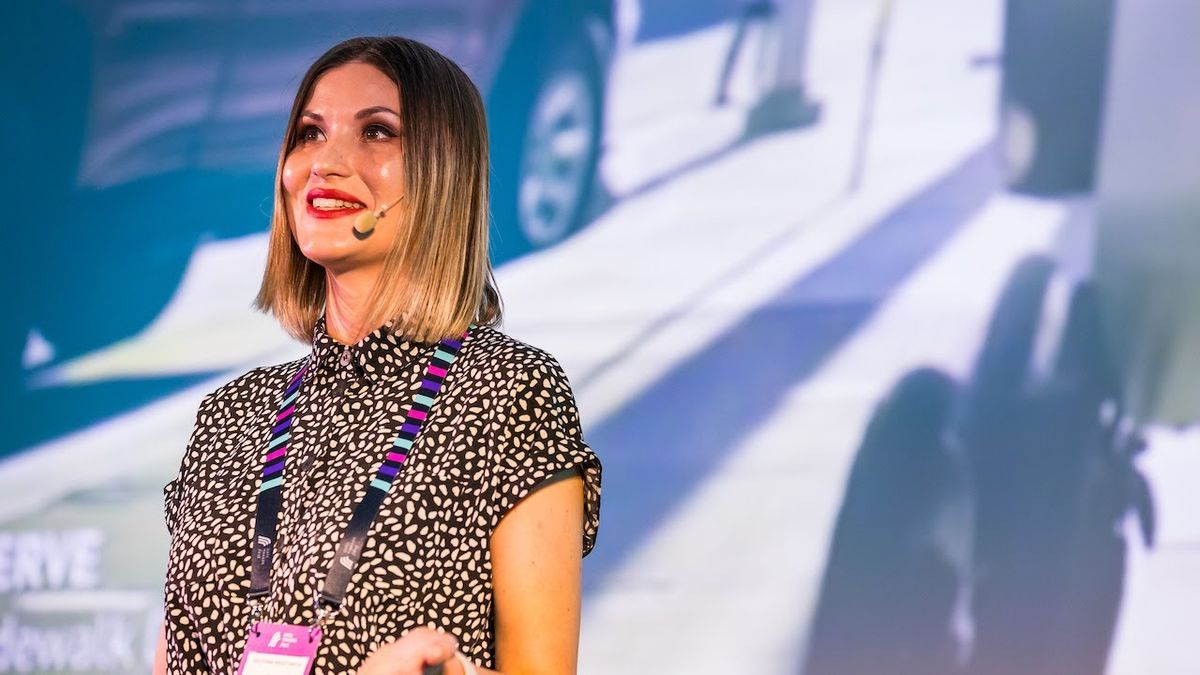
Synthetic data is annotated information that computer simulations or algorithms generate as an alternative to real world data. – Ekaterina S.
Collecting and annotating data is a time-consuming and expensive process, and to ensure models can generalize well, the data must be diverse and balanced. Recent advancements in simulation tools and generative models have led many computer vision AI practitioners to consider synthetic data as a possible alternative to real data. In this story, Ekaterina Sirazitdinova of NVIDIA discusses the benefits and challenges of synthetic data and will share a typical workflow of synthetic data creation.
Table of Contents
- The promise of synthetic data
- Challenges with real data
- Learning with synthetic data
- Addressing the ‘seem to real’ domain gap
- Testing and improving AI models
- The role of simulation tools and generative models
- Applications of synthetic data
- The power of automation
- The feedback loop in synthetic data
- The importance of diversity in synthetic data
- The versatility of robots
- The expensive nature of real data
The promise of synthetic data
Synthetic data, generated by computer simulations or algorithms, can potentially enable artificial general intelligence.
It offers numerous benefits for Robotics and perception applications, including cost savings, accuracy, and the ability to address corner cases and longtail anomalies.
Challenges with real data
Real data presents several challenges, including difficulties in labeling, collecting, and annotating data, especially for complex tasks like semantic segmentation and 3D point cloud processing.
Synthetic data can address these challenges by providing ground truths for situations where humans struggle to label, incorporating indirect features, and offering full programmability.