The True Cost of Compute in AI | a16z Podcast
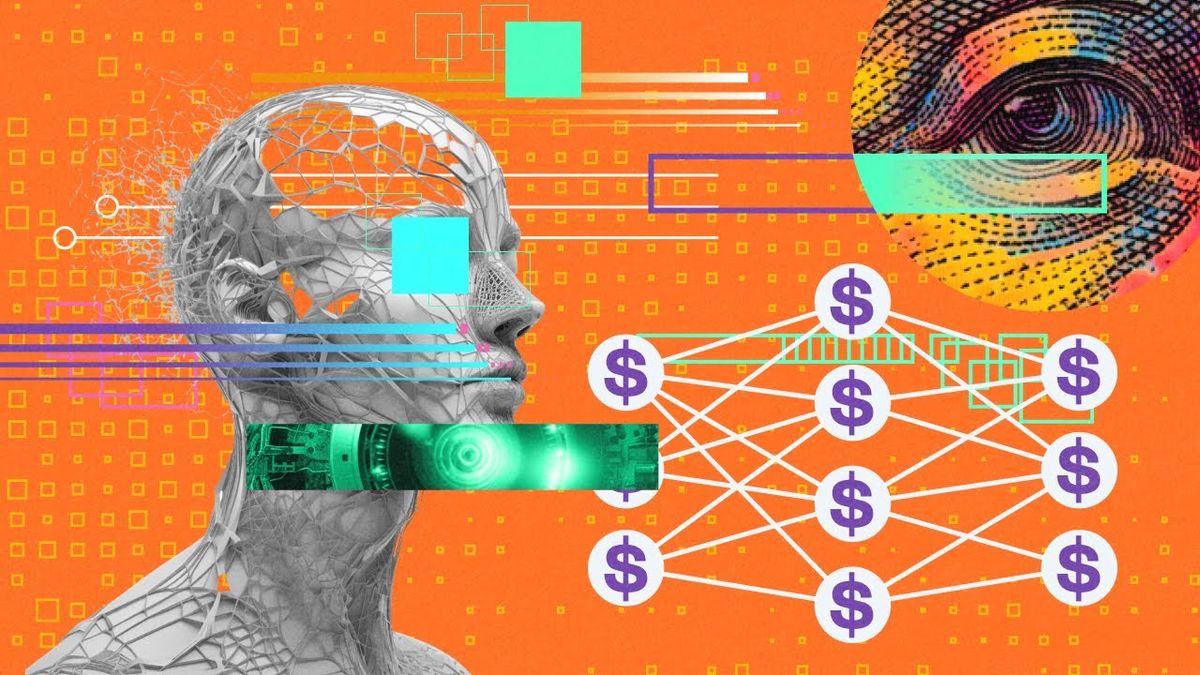
In the final segment of the AI hardware series, the podcast delves into the true cost of computing, particularly in the context of AI.
As the world generates more data, the need for faster and more resilient hardware becomes more critical.
The discussion focuses on the cost of training AI models, the implications of these costs, and the future of AI development in light of these costs.
The High Cost of AI Model Training
Training large language models can cost millions of dollars, which is a significant part of many AI companies’ capital expenditure.
In fact, some companies spend more than 80% of their total capital raised on compute resources.
This cost is a critical factor in the success of AI companies, irrespective of their size.
The Inefficiency of Less Performance Chips
Piecing together less performance chips is inefficient for model training and requires sophisticated software to manage.
Access to compute resources has become a determining factor for the success of AI companies and this is not just true for the largest companies building the largest models. In fact, many companies are spending more than 80 percent of their total capital raised on compute resources. – Podcast Narrator
The Barrier of Large Capital Investments
The cost for training these models may top out or even decrease as chips get faster and we don’t discover new training material as quickly.
The barrier to entry created by large capital investments is more of a speed bump than a significant obstacle.
Limited Availability of Training Material
Large models today already leverage a significant portion of all human knowledge in a particular area.
However, increasing the amount of data used by a factor of 100 may not be possible as we simply haven’t produced enough knowledge yet.
The Future of AI Innovation
With the cost of training large language models becoming more accessible for well-funded startups, more innovation in this area is expected in the future.
Factors Determining Training Costs
The cost of training an AI model depends on several factors, including batch size, learning rate, and the duration of the training process.
While some simplifications can be made, the reality of calculating these costs is quite complex.
The Expense of Large Models
Training large models like GPT3, which has about 175 billion parameters, can cost millions of dollars.
This high cost is due to the computational requirements for training the model and ultimately for inference.
The Cost of Inference
The cost of inference, or using the already trained model to elicit a response, is much cheaper than the cost of training.
However, it is still significant, especially when provisioning for peak capacity is considered.
Implications for AI Industry
The high cost of computing has implications for the AI industry.
Heavily capitalized incumbents have an advantage due to their ability to afford the high cost of computing, which often leads to better models.
Training one of these large language models today is not a hundred thousand dollar thing, it’s probably millions of dollars thing. Practically speaking what we’re seeing in industry is that it’s actually more for tens of millions of dollars thing. – Guido Apenzeller
The Decreasing Cost of Training
Despite the high costs, the overall cost of training these models seems to be decreasing.
This is due in part to becoming data-limited, where the size of the model needs to correspond to the amount of available training data.
Training Large Models within Reach
Training a large language model is within reach for a well-funded startup today, and for this reason, more innovation in this area is expected in the future.
The Role of Hardware
Software is eating the world, but hardware is coming along for the ride.
Understanding the cost and implications of computing, particularly for AI model training, is increasingly important.
Comments ()