What makes large language models expensive?
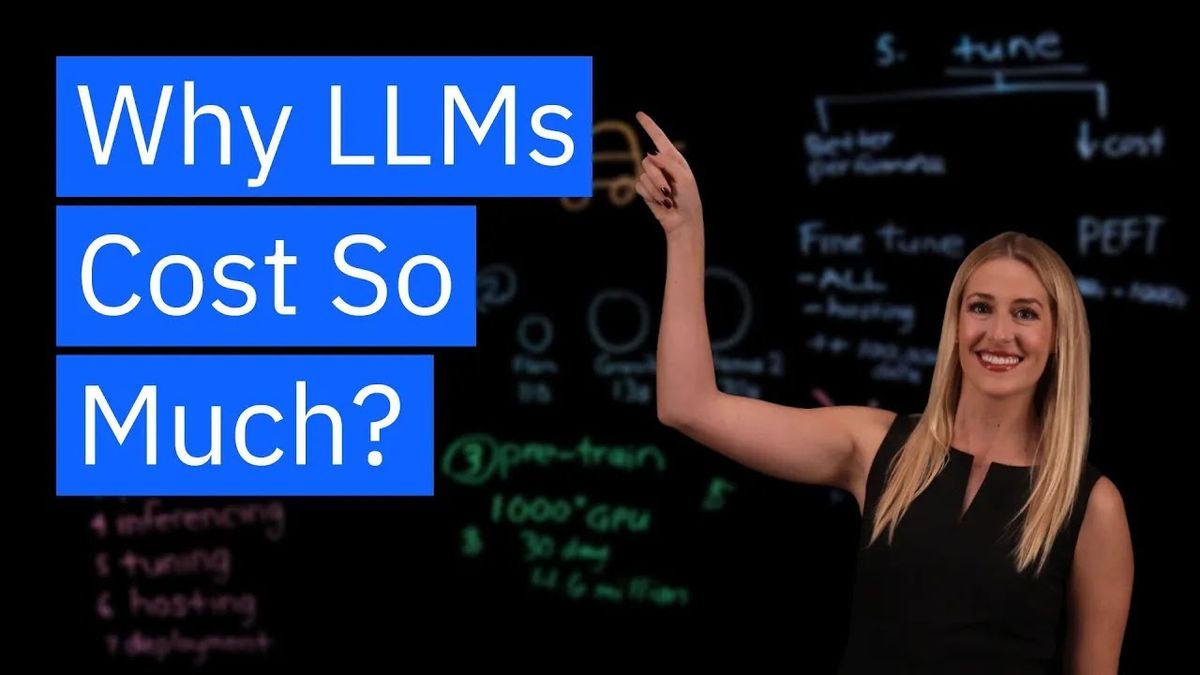
The larger the [AI] model is, the more parameters it has, and that’s going to drive compute and different resources. – Jessica Ridella
Jessica Ridella, IBM’s Global Sales Leader for the watsonx.ai generative AI platform, breaks down the pivotal factors that influence the cost of large language models in businesses.
She provides a comprehensive understanding of elements such as model size, use case, pre-training costs, inferencing, tuning, hosting costs and deployment options.
Table of Contents
- The Role of Use Case
- Model Size Impact
- Pre-Training Costs
- Understanding Inferencing Costs
- The Significance of Tuning
- Hosting Costs
- Deployment Options
The Role of Use Case
Different use cases necessitate different models and methods which require varying amounts of compute power.
It’s advisable to engage in a pilot with a partner or vendor to identify potential challenges and determine if generative AI is an appropriate solution.
Model Size Impact
The size and complexity of the generative AI model significantly affect pricing.
Larger models demand more computing resources.
Vendors often have pricing tiers based on model size, thus it’s crucial to discern whether they provide flexibility according to your specific use case.